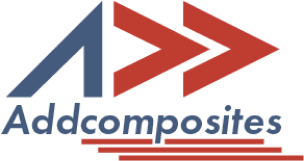
Automated Fiber Placement (AFP) is an additive composite manufacturing technique that has gained popularity in recent years due to its ability to produce complex geometries with high precision and efficiency. However, a pressing challenge facing this technology is defect detection and repair. Manual defect inspection is time-consuming and can be prone to errors, which can lead to costly rework or even part failure. To address this issue, researchers have been exploring automated methods of defect detection using machine learning techniques such as convolutional neural networks (CNNs). In this blog post, we will explore how CNNs can be used for automated defect detection in AFP and how the AddPath software's new defect detection feature can help streamline this process.
AddPath is a software platform that provides end-to-end solutions for composite manufacturing processes, including AFP. The software offers a range of features such as part design, toolpath generation, and process simulation. Recently, AddPath has updated its platform with a new feature that allows for automated defect detection in AFP using CNNs, incorporating both traditional and live mode options.
The updated defect detection feature in AddPath works by analyzing the AFP scan point cloud and images using a pre-trained CNN model. Users can either upload their scan data to the software or directly stream them from the AFP system running in live mode. The defect detection analysis can be run with just a few clicks, and the software then highlights any defects found in the scan point cloud, providing detailed information about their location, size, and type.
One of the key advantages of using AddPath's updated defect detection feature is its speed, accuracy, and real-time capabilities. The CNN model used by the software has been trained on large datasets of AFP scan images with labeled defects, which allows it to quickly and accurately identify defects in new scan images. This can save manufacturers significant time and resources compared to manual inspection methods. Moreover, the live mode option enables operators to monitor and address defects in near real-time, further enhancing the efficiency and quality of the manufacturing process.
Convolutional Neural Networks (CNNs) are a type of deep learning algorithm that are commonly used for image processing and computer vision tasks. CNNs are designed to automatically learn and extract features from images, which makes them well-suited for tasks such as object recognition, segmentation, and classification.
The basic architecture of a CNN consists of several layers, including convolutional layers, pooling layers, and fully connected layers. In the convolutional layer, the network applies a set of filters to the input image to extract features such as edges, corners, and textures. The pooling layer then downsamples the feature maps to reduce their size while preserving important information. Finally, the fully connected layer uses the extracted features to make predictions about the input image.
In automated defect detection for AFP, AddPath works with point cloud scans and then converts them into images. CNNs can be trained on large datasets of these converted AFP scan to images with labeled defects to learn how to identify different types of defects. Once trained, these models can be used to quickly and accurately detect defects in new scan images, both in traditional and live mode setups.
In the next section of this blog post, we will explore how AddPath's defect detection feature uses CNNs for automated defect detection in AFP. We will also provide step-by-step instructions for using this feature in AddPath, including the live mode option that allows for real-time defect detection and monitoring.
With the new update in AddPath, the software now allows users to directly stream scanned point cloud and related images from the AFP system running in live mode to the cloud server running the CNN model for inferencing. This enhancement improves the efficiency of the defect detection process and allows operators to monitor the predictions in near real time. To use AddPath's defect detection feature in live mode, follow these steps:
As the scanned point cloud and related images are streamed to the cloud server, the CNN model analyzes them and provides predictions for defects. The predictions are then streamed back to AddPath and displayed on the operator's screen in near real-time. This enables the operator to monitor the predicted defect values and give a go-ahead for the layer layup with AFP, ensuring a more efficient and accurate manufacturing process.
By utilizing AddPath's live mode for defect detection, manufacturers can quickly identify and address any issues in their AFP process before they become more serious problems. The ability to detect multiple types of defects simultaneously, coupled with valuable information about the defects' size and location, empowers operators to make informed decisions in real-time and maintain the highest quality standards.
Currently, AddPath's defect detection mode is in its beta stage and is being tested with trusted partners. These tests aim to evaluate the performance and accuracy of the defect detection feature before launching it for other players in the industry. Initial trial results demonstrate that AddPath's CNN model is highly accurate at detecting AFP defects, making it a valuable tool for manufacturers looking to improve their defect detection process.
In addition to its high accuracy, AddPath's defect detection feature is also fast and easy to use. The software can analyze the AFP process as it is ongoing, enabling real-time defect detection and monitoring. This feature can save manufacturers significant time compared to manual inspection methods, improving overall efficiency and reducing the likelihood of costly errors.
As the beta testing phase progresses and the defect detection feature proves to be effective with trusted partners, AddPath will prepare to launch the feature for a wider audience. This will allow more manufacturers to benefit from the advanced capabilities of AddPath's automated defect detection in their AFP processes, ensuring higher-quality composite manufacturing and a more streamlined workflow.
Automated defect detection with Convolutional Neural Networks (CNNs) significantly enhances the efficiency and accuracy of AFP manufacturing processes. By harnessing machine learning, manufacturers can swiftly and accurately identify defects, reducing costs, elevating quality, and boosting productivity.
AddPath's updated defect detection feature offers an intuitive platform for automated AFP defect detection, utilizing pre-trained CNN models for precise defect identification. With its high accuracy, speed, and user-friendly interface, the feature outperforms manual inspection methods in both efficiency and effectiveness.
In this blog post, we've delved into CNNs' functionality and their application in automated AFP defect detection. We've also guided you through using AddPath's defect detection feature and shared initial testing results. We trust that this post has illuminated the advantages of employing CNNs in AFP processes. To learn more about AddPath or schedule a demo, kindly visit our website or reach out to us directly.
Automated Fiber Placement (AFP) is an additive composite manufacturing technique that has gained popularity in recent years due to its ability to produce complex geometries with high precision and efficiency. However, a pressing challenge facing this technology is defect detection and repair. Manual defect inspection is time-consuming and can be prone to errors, which can lead to costly rework or even part failure. To address this issue, researchers have been exploring automated methods of defect detection using machine learning techniques such as convolutional neural networks (CNNs). In this blog post, we will explore how CNNs can be used for automated defect detection in AFP and how the AddPath software's new defect detection feature can help streamline this process.
AddPath is a software platform that provides end-to-end solutions for composite manufacturing processes, including AFP. The software offers a range of features such as part design, toolpath generation, and process simulation. Recently, AddPath has updated its platform with a new feature that allows for automated defect detection in AFP using CNNs, incorporating both traditional and live mode options.
The updated defect detection feature in AddPath works by analyzing the AFP scan point cloud and images using a pre-trained CNN model. Users can either upload their scan data to the software or directly stream them from the AFP system running in live mode. The defect detection analysis can be run with just a few clicks, and the software then highlights any defects found in the scan point cloud, providing detailed information about their location, size, and type.
One of the key advantages of using AddPath's updated defect detection feature is its speed, accuracy, and real-time capabilities. The CNN model used by the software has been trained on large datasets of AFP scan images with labeled defects, which allows it to quickly and accurately identify defects in new scan images. This can save manufacturers significant time and resources compared to manual inspection methods. Moreover, the live mode option enables operators to monitor and address defects in near real-time, further enhancing the efficiency and quality of the manufacturing process.
Convolutional Neural Networks (CNNs) are a type of deep learning algorithm that are commonly used for image processing and computer vision tasks. CNNs are designed to automatically learn and extract features from images, which makes them well-suited for tasks such as object recognition, segmentation, and classification.
The basic architecture of a CNN consists of several layers, including convolutional layers, pooling layers, and fully connected layers. In the convolutional layer, the network applies a set of filters to the input image to extract features such as edges, corners, and textures. The pooling layer then downsamples the feature maps to reduce their size while preserving important information. Finally, the fully connected layer uses the extracted features to make predictions about the input image.
In automated defect detection for AFP, AddPath works with point cloud scans and then converts them into images. CNNs can be trained on large datasets of these converted AFP scan to images with labeled defects to learn how to identify different types of defects. Once trained, these models can be used to quickly and accurately detect defects in new scan images, both in traditional and live mode setups.
In the next section of this blog post, we will explore how AddPath's defect detection feature uses CNNs for automated defect detection in AFP. We will also provide step-by-step instructions for using this feature in AddPath, including the live mode option that allows for real-time defect detection and monitoring.
With the new update in AddPath, the software now allows users to directly stream scanned point cloud and related images from the AFP system running in live mode to the cloud server running the CNN model for inferencing. This enhancement improves the efficiency of the defect detection process and allows operators to monitor the predictions in near real time. To use AddPath's defect detection feature in live mode, follow these steps:
As the scanned point cloud and related images are streamed to the cloud server, the CNN model analyzes them and provides predictions for defects. The predictions are then streamed back to AddPath and displayed on the operator's screen in near real-time. This enables the operator to monitor the predicted defect values and give a go-ahead for the layer layup with AFP, ensuring a more efficient and accurate manufacturing process.
By utilizing AddPath's live mode for defect detection, manufacturers can quickly identify and address any issues in their AFP process before they become more serious problems. The ability to detect multiple types of defects simultaneously, coupled with valuable information about the defects' size and location, empowers operators to make informed decisions in real-time and maintain the highest quality standards.
Currently, AddPath's defect detection mode is in its beta stage and is being tested with trusted partners. These tests aim to evaluate the performance and accuracy of the defect detection feature before launching it for other players in the industry. Initial trial results demonstrate that AddPath's CNN model is highly accurate at detecting AFP defects, making it a valuable tool for manufacturers looking to improve their defect detection process.
In addition to its high accuracy, AddPath's defect detection feature is also fast and easy to use. The software can analyze the AFP process as it is ongoing, enabling real-time defect detection and monitoring. This feature can save manufacturers significant time compared to manual inspection methods, improving overall efficiency and reducing the likelihood of costly errors.
As the beta testing phase progresses and the defect detection feature proves to be effective with trusted partners, AddPath will prepare to launch the feature for a wider audience. This will allow more manufacturers to benefit from the advanced capabilities of AddPath's automated defect detection in their AFP processes, ensuring higher-quality composite manufacturing and a more streamlined workflow.
Automated defect detection with Convolutional Neural Networks (CNNs) significantly enhances the efficiency and accuracy of AFP manufacturing processes. By harnessing machine learning, manufacturers can swiftly and accurately identify defects, reducing costs, elevating quality, and boosting productivity.
AddPath's updated defect detection feature offers an intuitive platform for automated AFP defect detection, utilizing pre-trained CNN models for precise defect identification. With its high accuracy, speed, and user-friendly interface, the feature outperforms manual inspection methods in both efficiency and effectiveness.
In this blog post, we've delved into CNNs' functionality and their application in automated AFP defect detection. We've also guided you through using AddPath's defect detection feature and shared initial testing results. We trust that this post has illuminated the advantages of employing CNNs in AFP processes. To learn more about AddPath or schedule a demo, kindly visit our website or reach out to us directly.